Keynote Speakers
Classification by Association
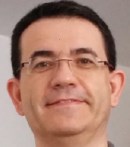
Elements of musical intelligence for the next generation of digital musical tools
Musical intelligence can be observed in many diverse situations: from tapping to music to the recommendation of interesting (and probably unknown) tracks. In the last decades we have improved our knowledge about music perception and cognition, and our skills to build artificial systems that apparently behave with some musical intelligence. In this talk I will address different aspects of what could be considered "musical intelligence" in humans, and how our current music technologies mock some of them, fail at simulating others, or (sometimes) do something different but interesting. In this context some of the outcomes of a recently finished EU project, GiantSteps, addressed to study and develop intelligent software components in music creation scenarios, will be presented.
Perfecto Herrera holds a B.S. in Psychology and is with the Music Technology Group, Universitat Pompeu Fabra, Barcelona since 1997. There, as researcher and project manager, he contributed to the MPEG-7 standardization initiative from 1999 to 2001 and to the EU-IST-funded projects CUIDADO (2000-2002), Semantic Interaction with Music Audio Contents (2003-2005), EMergent Cognition for Active Perception (2006-2008) and GiantSteps (2013-2016). He was (2005-2011) the Director of the Department of Sonology, Superior Music School of Catalonia (ESMUC), where he teaches Music Technology, Acoustics, Psychoacoustics, Critical Listening Skills, and Technologies for Music Research. His main research interests are audio and music content processing, classification in humans and machines, and music perception, cognition and emotion, areas in which he has co-authored more than 150 research papers and articles in peer-reviewed international conferences and journals.
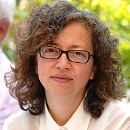
Abductive Reasoning on Compliance: a balance between flexibility and regulation
Many emerging applications in Business Processes, Clinical Guidelines, Service-Oriented and Multi-Agent Systems are characterized by distribution, complex interaction and coordination dynamics.
Such domains, apparently unrelated, usually ask for a high level of flexibility, thus making a complete, rigid and detailed model of interaction not suitable to their representation. On the other hand, interaction must be disciplined so as to ensure that involved entities comply with regulations, norms, business rules, protocols, as well as time and data constraints. Practically speaking, a good balance between flexibility and regulation is needed.
In this talk, I will introduce how methods, tools and techniques of Computational Logic, and Abductive Reasoning in particular, can be fruitfully adopted to model and reason on compliance in such emerging scenarios providing that required balance.
Thanks to the formalization of declarative interaction models and their dynamics in terms of Constraint Abductive Logic Programming, run-time reasoning on compliance – even in presence of constraints on time and data, and incompleteness of the knowledge – becomes flexible, concrete and effective.
Paola Mello is currently Full Professor at the Department of Computer Science and Engineering of the University of Bologna, where she teaches Artificial Intelligence and Foundations of Computer Science. From 2010 to 2014 she has been the President of the Italian Association for Artificial Intelligence. From 2012 to 2015 she has been the Head of the Department of Computer Science and Engineering of the University of Bologna.
Paola Mello’s research focuses on artificial intelligence, particularly on knowledge representation and reasoning, computational logic, logic programming, multi-agent systems, decision support systems and machine learning, with applications in different areas (e.g. e-Health). She has also contributed to novel algorithms and reasoning tools, which have been applied to domains involving complex, distributed and heterogeneous processes, such as Web services orchestrations, data-aware business processes, socio-technical systems, and computerized clinical guidelines. Moreover, she has contributed to cutting-edge technology for run-time and a-posteriori verification, used to monitor process conformance with respect to regulations, standards and protocols.
Her research has covered implementation, application and theoretical aspects, and she is author of several national and international publications. She took part in program committees of national and international workshops and conferences and in several national and international (UE) research projects in collaboration with Universities and Research Centers worldwide.
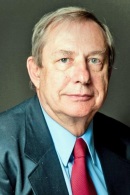
Security of Cyber-Physical Systems
Cyberspace, the ubiquitous collection of interconnected IP networks and hosts, has become the
nervous system of the country. Healthy functioning of Cyberspace is essential for the proper
operation of numerous critical infrastructures, including telecommunication, energy distribution,
and transportation. It is also necessary to support the ever expanding business infrastructure for
commerce and banking. The increasing reliance on Cyberspace has been paralleled by a
corresponding increase in the variety, frequency and impact of attacks from a range of assailants.
Both commercial companies and government agencies face continuous and increasingly more
sophisticated cyber-attacks ranging from data exfiltration and spear phishing to sophisticated
worms and logic bombs. The targets include not only computer information systems, but also the
network communication infrastructure and power grids.
In this talk, I will discuss protecting cyber-physical systems from attacks and argue that cyber
security can significantly benefit from multidisciplinary research including Web Intelligence, Data
Analytics and Network Science.
Marek Rusinkiewicz is a computer scientist, an educator, and a
former research executive. Currently he is the Dean Emeritus of the
Ying Wu College of Computing at the New Jersey Institute of
Technology. Previously he was a Senior Group Vice President and
the General Manager of Applied Research Laboratories at Telcordia
Technologies (formerly Bell Communication Research), which
included R&D centers in New Jersey, Texas, Taiwan and Poland.
Before joining Telcordia, Rusinkiewicz was the Vice President for
Information Technology Research at the Microelectronics and
Computer Technology Corporation (MCC) in Austin, a leading
industrial R&D consortium, where he led a number of initiatives
aimed at the development of next generation information
management technologies, including web search, semantic agents,
and collaboration management.
Rusinkiewicz has held academic positions at the University of Glasgow, the University of
Michigan, and the University of Houston, where he was a Professor of Computer Science until
1999. His research interests include heterogeneous database systems, workflow management,
agent-based systems and cyber security. In 2006-2010 he was a member President’s Council of
Advisors on Science and Technology (PCAST) Networking and Information Technology
Technical Advisory Group.
He is Editor-in-Chief of the World Wide Web Journal and serves on advisory boards of several
Universities and Research Centers. He has consulted for numerous industry and government
organizations in the USA, Japan, China and Europe.
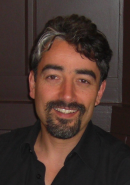
Classification by Association
In machine learning the centre of attention these days is on Deep Learning. The spotlight has turned towards this re-branding of Neural Networks because of the tremendous success it recently demonstrated for image classification, speech recognition, text categorization and other applications where labeled data is plentiful. However, this is not necessarily true when data is not sufficient enough to optimize the hyperparameters. Alternatives are still needed in most applications and we advocate for rule-based classifiers such as associative classifiers. Associative classifiers piggyback on association rule mining to discover classification rules. The learned model is a set of rules that are easy to understand and can be edited. Established associative classification algorithms have shown to be very effective in handling categorical data such as text data. There were many improvements this last decade for rule representation, rule pruning, rule selection strategies, etc. Yet, most proposed approaches still suffer from the limitations of the support-confidence framework inherited from association rule mining. We will talk about the challenges in mining these classification rules, the progress reached using this paradigm and the last incarnation of associative classifiers that finds statistical dependency in the data to avoid the detection of meaningless rules and the omission of interesting ones while doing away with the restraining parameters support and confidence.
Osmar R. Zaïane is a Professor in Computing Science at the University of Alberta, Canada, and Scientific Director of the Alberta Innovates Centre for Machine Learning (AICML). Dr. Zaiane obtained his Ph.D. from Simon Fraser University, Canada, in 1999. He has published more than 200 papers in refereed international conferences and journals. He is Associate Editor of many International Journals on data mining and data analytics and served as program chair and general chair for scores of international confreences in the field of knowledge discovery and data mining. Dr. Zaiane received numerous awards including the 2010 ACM SIGKDD Service Award from the ACM Special Interest Group on Data Mining, which runs the world’s premier data science, big data, and data mining association and conference.